AI/ML in Business: Developing a Strategy for 2024
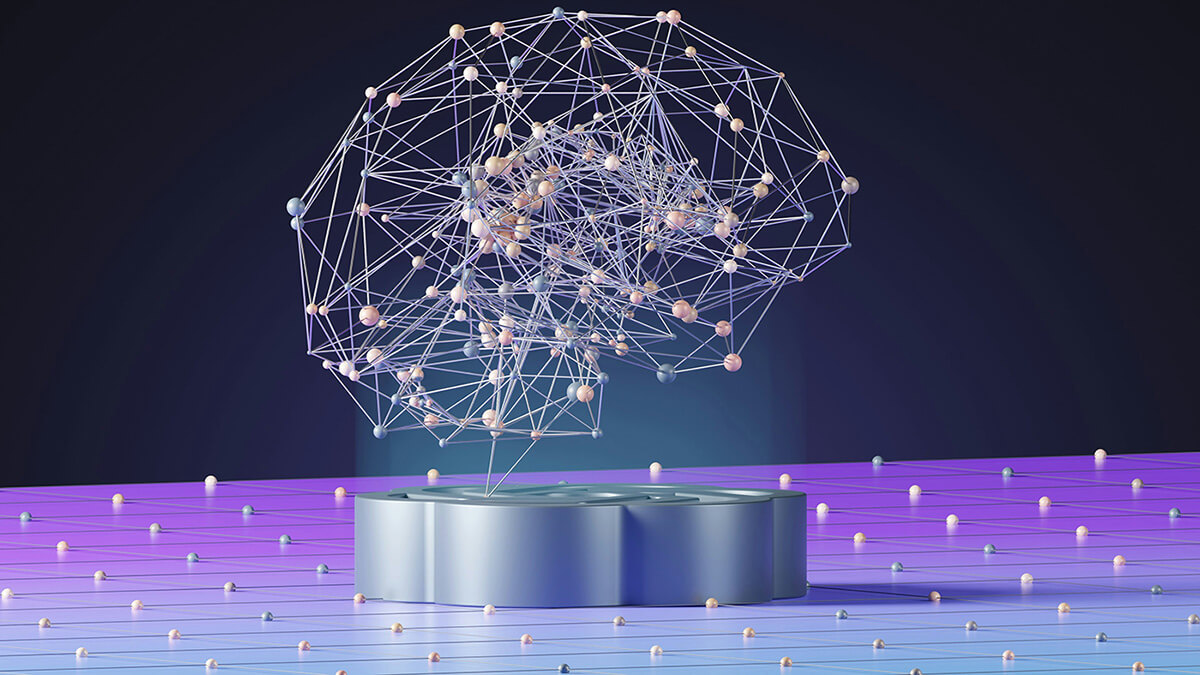
Gartner predicts that by 2026, in an enterprise setting, more than 100 million individuals will be actively engaging with colleagues who exist in the virtual space. Looking further ahead, the introduction of AI in business to augment or autonomously perform tasks, certain activities, or even entire jobs is expected to lead to the appearance of over 500 million net new human jobs.
This projection challenges the often-discussed notion of automation leading to widespread job displacement, and highlights the potential for AI technologies to act as catalysts for job creation and evolution. As Deloitte mentions in its State of AI in the Enterprise, 4th Edition report, 60% of high-achieving companies have a full-fledged AI strategy in place. In this article, we share insights to help you understand the particulars of embracing AI and ML in business and start designing strategies of your own.
Future-Ready Technical Approaches with AI and ML
Implementing Advanced Machine Learning Models
Successfully implementing advanced Machine Learning (ML) models within a business context begins with a clear understanding and definition of specific objectives that ML aims to address. To do that, establish guidelines for the use of ML within the organization, keeping in mind ethical considerations. To set the stage for a holistic approach, a sound idea is to build a cross-functional team of diverse members such as data scientists, data engineers, domain experts, and business analysts to foster collaboration and effective communication.
Developing a robust ML strategy is a crucial next step, encompassing considerations for data quality, privacy, and security. Simultaneously, you should establish data governance practices to lay the groundwork for successful ML implementation. The evaluation of infrastructure and the technology stack, including the exploration of scalable cloud platforms, is imperative to ensure the necessary technical foundation for ML workflows.
You must also conduct a thorough return on investment (ROI) analysis to validate if the anticipated benefits align with broader business expectations. Moreover, staying informed about and compliant with relevant data protection and privacy regulations is critical in light of the rapid ML evolution. Engage in finding ways to identify and mitigate potential risks associated with ML implementation, along with the development of contingency plans for unforeseen challenges.
As the implementation progresses, planning for scalability and the seamless integration of ML models into existing business processes will become your ongoing considerations. Implementing systems for continuous monitoring, iteration, and improvement based on feedback and evolving business needs will help you ensure the adaptability and relevance of ML solutions over time.
Let’s not forget that education also plays a pivotal role in the successful integration of ML into business operations. This involves not only enlightening non-technical stakeholders on the value and implications of ML but also instilling a culture of data-driven decision-making across the organization. At the same time, initiating a small-scale Proof-of-Concept (PoC) will help you showcase the feasibility and potential impact of an ML solution.
Last but not least, well-defined communication—including engagement with industry leaders, attendance at conferences, and staying informed about emerging trends—will help you form a proactive approach and make the most of your Machine Learning strategy. If you manage to recognize and celebrate successful ML implementations while learning from failures and adapting accordingly, you will eventually solidify your organization’s commitment to innovation and improvement.
Strategic Deployment of AI in Business
The productivity scene is undergoing a transformative shift with the integration of AI that can be seamlessly deployed or built into applications to enhance and facilitate various tasks. Harvard Business Review shares a standout example of GitHub’s Copilot, which, within just two years since its inception, is responsible for writing a substantial 46% of the code on its repository. Notably, developers leveraging Copilot report a remarkable 55% increase in coding speed, freeing up valuable time for more creative pursuits. The impact extends beyond mere efficiency; three out of four users attest to its ability to save mental energy, allowing them to focus on more rewarding aspects of their work, such as creating novel solutions to new problems.
The application of AI in business workflows is a compelling proposition. Processes like payroll, onboarding, and IT help desk support, characterized by their repeatability and rules-based nature, can undergo significant streamlining through AI integration, HBR says. This trend has given rise to an entire category of AI software designed to handle manual tasks and optimize many business processes, promising increased efficiency and reduced operational friction.
A unique perspective on AI’s role in productivity involves its impact on time, particularly in fields like fraud detection and security analysis. Here, time emerges as a critical asset, and the ability to shorten the duration required to sift through vast amounts of data-rich and time-sensitive information becomes a defining factor for success. In such scenarios, HBR believes that AI not only enhances efficiency but becomes a strategic advantage, enabling professionals to be more effective in their roles.
In essence, the evolving landscape of AI in productivity is not just about doing things faster but fundamentally changing how tasks are approached and executed. It’s about empowering individuals to focus on the creative and intellectually stimulating aspects of their work, driving innovation, and reshaping traditional business processes for greater agility and effectiveness.
Harnessing Predictive Analytics for Technical Resilience
By delving into historical data, AI/ML models discern patterns that indicate normal system behavior, enabling you to spot anomalies and potential issues early on. This proactive stance extends to anticipating and addressing technical problems before they escalate, meaning you can minimize downtime and elevate overall system resilience. Capacity planning becomes more strategic as organizations leverage predictive analytics to forecast future resource needs, facilitating efficient maintenance scheduling for optimal performance.
The risk assessment capabilities inherent in AI/ML models contribute significantly to prioritizing efforts aimed at enhancing resilience. This involves a multifaceted consideration of factors such as software vulnerabilities, hardware reliability, and ever-evolving cybersecurity threats. AI’s adaptive security measures continuously learn and evolve, staying attuned to emerging threats and contributing to a dynamic defense strategy.
In the event of a technical failure, predictive analytics steps in with rapid root cause analysis, streamlining your company’s incident response and mitigating the impact on its operations. The goal is to encourage data-driven decision-making, giving you an opportunity to make informed choices on tech investments and improvements. This approach ensures a continuous learning and adaptation cycle, positioning your organization to navigate complexities with resilience and agility.
Integrating AI for Real-Time Decision Support
Additionally, the integration of AI in business emerges as a pivotal force, promising to reshape decision-making paradigms. Transparency takes center stage as the focus shifts towards understanding the intricacies of AI predictions—a key element in establishing trust in the decision-making process.
According to one of McKinsey’s Inside the Strategy Room podcast episodes, the journey of AI in decision-making unfolds progressively, starting with advisory roles for executives and culminating in the prospect of fully autonomous decision-making. McKinsey underscores, however, that the benefits of AI might vary for companies, with those reliant on a few major decisions or facing volatility possibly reaping less reward. Yet, even in the face of uncertainty, AI can be leveraged to predict external events and enhance control.
The speed of decision-making becomes a critical factor, with AI proving most valuable when decisions require real-time adjustments close to resource deployment. The distinction between strategic and tactical decisions comes into play, highlighting that seemingly tactical uses of AI for business intelligence, such as scanning for patterns in consumer behavior, can yield substantial strategic advantages.
The McKinsey podcast portrays AI as a neutral ally, offering a systematic and data-driven foundation for managing debates and supporting decision-makers. The AI also assumes the role of a guiding force, reminding decision-makers of their original intentions amid short-term pressures. In essence, AI in business emerges not as a replacement for decision-makers but as a valuable augmentation, providing insights and nudges that contribute to more informed, dynamic, and consistent strategic choices.
Innovative AI Strategies for Technical Excellence
In the pursuit of technical excellence, organizations are embracing innovative AI strategies that extend beyond conventional applications. A crucial element of this strategy involves actively seeking and integrating new AI solutions tailored to specific domains and operational areas. For example, in project management, it could be meeting note-taking, where automated systems can extract key insights from discussions, enhancing efficiency and facilitating a more seamless workflow.
In the creative content production field, AI and ML are making significant strides, as seen with technologies like DALL·E, Midjourney, and Stable Diffusion. These innovations, capable of generating diverse and contextually relevant images from textual descriptions, are redefining the standards of visual representation. Sectors heavily reliant on imagery, such as marketing and design, can leverage such cutting-edge AI/ML solutions to elevate technical excellence and creativity. With tools like PIKA, video generation is another frontier where AI is making waves. This technology, harnessing AI for video creation, presents a paradigm shift in content production. From marketing campaigns to educational materials, integrating these AI-driven solutions not only streamlines the process but also introduces a level of creativity and customization that was previously challenging to achieve.
Beyond these examples, industries are exploring AI solutions for specific tasks that align with their operational needs. In healthcare, AI algorithms for medical image analysis are enhancing diagnostic capabilities. In finance, AI-driven fraud detection systems are transforming security protocols. As your organization navigates the landscape of technical excellence, the integration of diverse AI solutions tailored to specific domains becomes paramount. The future of technical excellence lies in the proactive exploration and integration of cutting-edge solutions that align with industry-specific challenges and objectives.
A Deep Dive into AI and ML Adoption
Risks
The all-out adoption of Artificial Intelligence and Machine Learning in business brings about transformative benefits as well as risks. You must comprehend these risks and take steps to mitigate them for responsible AI deployment.
One major concern revolves around bias in AI models. If the training data is not diverse or representative, the AI model may even deepen existing societal biases, leading to discriminatory outcomes. Added to that, ethical considerations surrounding job displacement and economic inequality emerge as societal risks. As automation through AI/ML becomes more prevalent, certain jobs may become obsolete, potentially widening economic disparities (although Gartner’s findings mentioned at the beginning suggest otherwise). This means that balancing the benefits of automation with strategies for workforce transition and upskilling is essential.
Security vulnerabilities present a serious risk, particularly as AI systems become part and parcel of critical infrastructure. Adversarial attacks, where malicious actors manipulate inputs to deceive AI models, can compromise the integrity and reliability of AI systems. Ensuring robust cybersecurity measures is critical to prevent unauthorized access and manipulation of AI algorithms. AI’s reliance on data also introduces privacy concerns. The collection and use of vast amounts of personal data for training raises ethical questions about consent and data ownership. While striking a balance between extracting valuable insights and protecting individual privacy is a persistent challenge, legal and regulatory risks are also on the horizon. The evolving nature of AI technology makes it challenging for laws and regulations to keep pace. Issues related to liability, accountability, and determining responsibility in case of AI-related incidents are areas that require careful legal consideration.
Transparency and interpretability pose another significant risk. Complex models, especially in deep learning, are often considered “black boxes,” meaning it is quite unclear how they arrive at particular decisions. Lack of transparency can hinder accountability and make it difficult to address errors or biases in the system. Moreover, overreliance on AI without human oversight poses operational risks. AI systems may fail to account for contextual nuances or unexpected scenarios, leading to incorrect decisions. Human oversight is crucial for intervention when AI systems make errors and ensuring that decisions align with ethical and legal standards.
While AI and ML offer tremendous potential, it is crucial to acknowledge the risks that accompany these technologies and address those risks promptly and adequately. To sum up, strategies for responsible AI deployment include:
- Ensuring unbiased and transparent models;
- Enhancing cybersecurity measures;
- Addressing privacy concerns;
- Maintaining human oversight;
- Navigating the legal landscape.
Remember: A comprehensive and proactive approach to mitigating these risks is essential for fostering the responsible and ethical use of AI regardless of the industry your organization operates in.
Tips
Gartner has come up with a great formula for AI adoption, which can also be easily applied to ML. It says that when it comes to integrating AI/ML technologies into operations, line-of-business stakeholders play a pivotal role in ensuring clarity and feasibility. To articulate tangible business benefits, stakeholders should address fundamental questions. These include identifying the specific business problem being addressed, determining the primary consumer of the technology, specifying the business processes that will host the Artificial Intelligence or Machine Learning techniques, enlisting subject matter experts from relevant lines of business to guide solution development, and establishing metrics for measuring the impact and ongoing value of the AI implementation. Monitoring and maintenance responsibilities are also crucial considerations and should be clearly defined.
Source: https://www.gartner.com/en/information-technology/topics/ai-strategy-for-business
Gartner’s feasibility criteria encompass multiple dimensions. On the technical front, an evaluation of existing technology options is essential, assessing how well they can elevate the stated business use case to a so-called “state-of-the-art” level. Internally, factors such as organizational culture, leadership support, stakeholder buy-in, skill availability, and ethical considerations are of great importance. Externally, feasibility spans regulatory compliance, social acceptance, and the compatibility of external infrastructure. This comprehensive approach ensures that the integration of AI/ML aligns not only with technical capabilities but also with the internal culture and external factors that influence successful implementation and sustained value.
AI and ML for Future-Proofing Your Business
Nowadays, we enjoy unprecedented access to customer insights, and our ability to understand their behavior has reached new heights. Leveraging our team’s profound expertise in Big Data and drawing from extensive cross-industry experience, we at Vodworks are at the forefront of deciphering customer preferences, tracking their journeys, analyzing clicks, understanding purchase patterns, and identifying points where engagement may drop.
This capability is made possible through the integration of cutting-edge Artificial Intelligence and Machine Learning technologies. By seamlessly combining data analytics and advanced algorithms, we not only gain valuable insights into customer behavior but also empower businesses to make informed decisions, refine strategies, and deliver tailored experiences that resonate with their audiences.
If you’re interested in unlocking the true potential of AI in business, making your data actionable, or building an AI/ML-enabled application from scratch, you’re more than welcome to reach out!
Talent Shortage Holding You Back? Scale Fast With Us
Frequently Asked Questions
In what industries can Web3 technology be implemented?
Web3 technology finds applications across various industries. In Retail marketing Web3 can help create engaging experiences with interactive gamification and collaborative loyalty. Within improving online streaming security Web3 technologies help safeguard content with digital subscription rights, control access, and provide global reach. Web3 Gaming is another direction of using this technology to reshape in-game interactions, monetize with tradable assets, and foster active participation in the gaming community. These are just some examples of where web3 technology makes sense however there will of course be use cases where it doesn’t. Contact us to learn more.
How do you handle different time zones?
With a team of 150+ expert developers situated across 5 Global Development Centers and 10+ countries, we seamlessly navigate diverse timezones. This gives us the flexibility to support clients efficiently, aligning with their unique schedules and preferred work styles. No matter the timezone, we ensure that our services meet the specific needs and expectations of the project, fostering a collaborative and responsive partnership.
What levels of support do you offer?
We provide comprehensive technical assistance for applications, providing Level 2 and Level 3 support. Within our services, we continuously oversee your applications 24/7, establishing alerts and triggers at vulnerable points to promptly resolve emerging issues. Our team of experts assumes responsibility for alarm management, overseas fundamental technical tasks such as server management, and takes an active role in application development to address security fixes within specified SLAs to ensure support for your operations. In addition, we provide flexible warranty periods on the completion of your project, ensuring ongoing support and satisfaction with our delivered solutions.
Who owns the IP of my application code/will I own the source code?
As our client, you retain full ownership of the source code, ensuring that you have the autonomy and control over your intellectual property throughout and beyond the development process.
How do you manage and accommodate change requests in software development?
We seamlessly handle and accommodate change requests in our software development process through our adoption of the Agile methodology. We use flexible approaches that best align with each unique project and the client's working style. With a commitment to adaptability, our dedicated team is structured to be highly flexible, ensuring that change requests are efficiently managed, integrated, and implemented without compromising the quality of deliverables.
What is the estimated timeline for creating a Minimum Viable Product (MVP)?
The timeline for creating a Minimum Viable Product (MVP) can vary significantly depending on the complexity of the product and the specific requirements of the project. In total, the timeline for creating an MVP can range from around 3 to 9 months, including such stages as Planning, Market Research, Design, Development, Testing, Feedback and Launch.
Do you provide Proof of Concepts (PoCs) during software development?
Yes, we offer Proof of Concepts (PoCs) as part of our software development services. With a proven track record of assisting over 70 companies, our team has successfully built PoCs that have secured initial funding of $10Mn+. Our team helps business owners and units validate their idea, rapidly building a solution you can show in hand. From visual to functional prototypes, we help explore new opportunities with confidence.
Are we able to vet the developers before we take them on-board?
When augmenting your team with our developers, you have the ability to meticulously vet candidates before onboarding. \n\n We ask clients to provide us with a required developer’s profile with needed skills and tech knowledge to guarantee our staff possess the expertise needed to contribute effectively to your software development projects. You have the flexibility to conduct interviews, and assess both developers’ soft skills and hard skills, ensuring a seamless alignment with your project requirements.
Is on-demand developer availability among your offerings in software development?
We provide you with on-demand engineers whether you need additional resources for ongoing projects or specific expertise, without the overhead or complication of traditional hiring processes within our staff augmentation service.
Do you collaborate with startups for software development projects?
Yes, our expert team collaborates closely with startups, helping them navigate the technical landscape, build scalable and market-ready software, and bring their vision to life.
Our startup software development services & solutions:
- MVP & Rapid POC's
- Investment & Incubation
- Mobile & Web App Development
- Team Augmentation
- Project Rescue
Subscribe to our blog
Related Posts
Get in Touch with us
Thank You!
Thank you for contacting us, we will get back to you as soon as possible.
Our Next Steps
- Our team reaches out to you within one business day
- We begin with an initial conversation to understand your needs
- Our analysts and developers evaluate the scope and propose a path forward
- We initiate the project, working towards successful software delivery